Clustering Analysis of Differentially Expressed Proteins
To verify the rationality and accuracy of the selected differential expression proteins or characteristic differential expression proteins, cluster analysis can be used to group proteins based on their expression trends, allowing for more intuitive proteomic data analysis.
1. Hierarchical Clustering Analysis
Perform hierarchical clustering analysis on the selected differential expression proteins, clustering proteins with the same or similar expression patterns. The clustering results of the differential expression proteins are shown in the figure below:
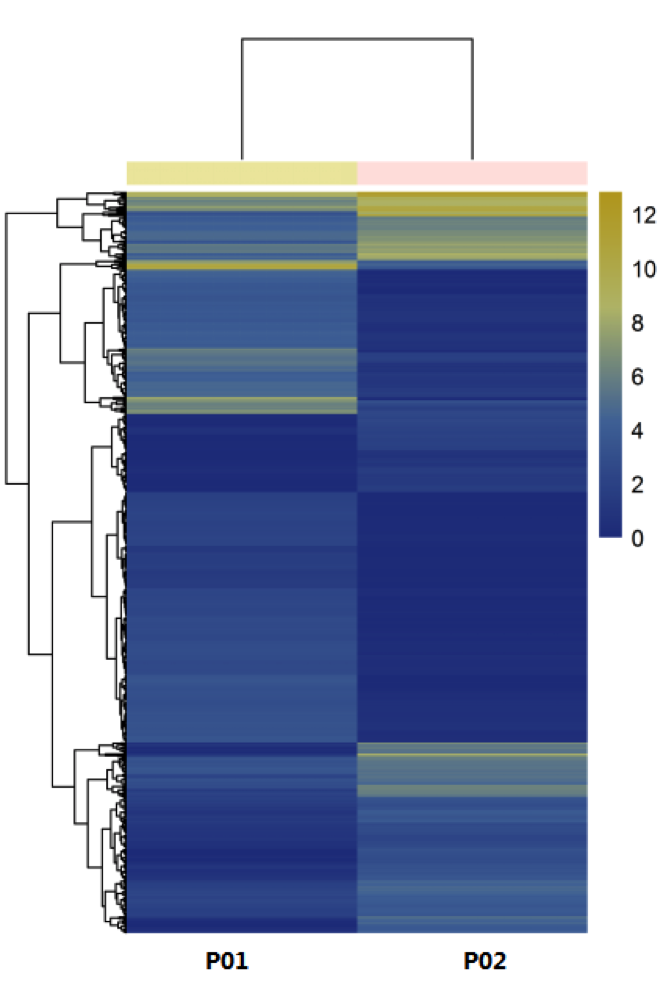
Figure 1 Hierarchical Clustering of Differential Expression Proteins
Note: Different columns in the figure represent different samples, and different rows represent different proteins. The colors represent the expression levels of proteins in the samples.
2. K-means Clustering Analysis
To study the expression patterns of proteins under different experimental conditions, K-means clustering analysis is performed on the expression levels of the samples. Proteins in the same cluster exhibit similar change trends under different experimental treatments, and proteins with similar change trends often have similar functions.
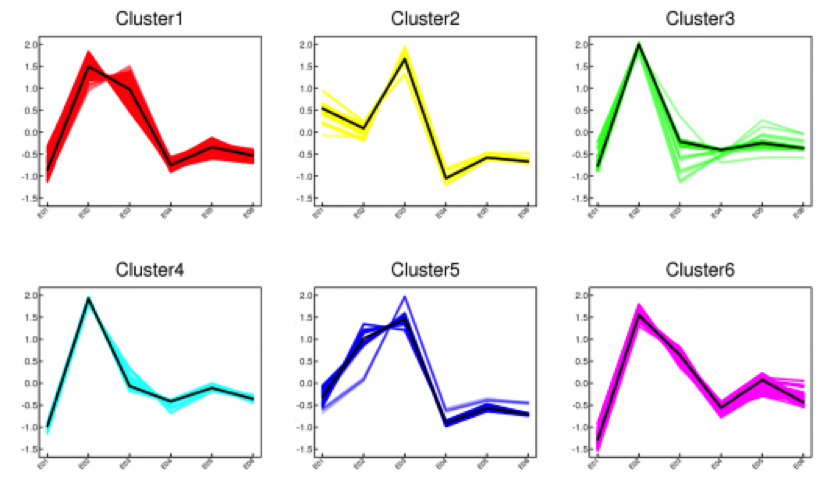
Figure 2 Line Chart of Differential Expression Protein Clustering
Note: The x-axis represents sample groups, and the y-axis represents expression levels. The black line represents a line chart of the average relative expression levels of all proteins in this cluster under different experimental conditions.
How to order?